Predicting Heart Failure Admission With CMR-Based Phenotype and Electronic Health Information
< Back To FeedHeart failure (HF) is a large and growing problem affecting an estimated 6.7M people in the US and more than 64M worldwide1,2. Healthcare systems are justifiably concerned about the cost of heart failure, projected to reach $69.8 billion annually in the US alone by 20301.
This study3 by Cornhill et al., from the precision medicine research group led by Cohesic CMO and co-founder Dr. James White, demonstrates the potential of combining patient-reported health status, data derived from Cardiovascular Magnetic Resonance (CMR) imaging, and electronic health information. Using data collected from 1,775 patients with chronic systolic HF, they developed a predictive model to identify which patients are at high risk of HF hospitalization and predict their time to hospitalization. Both a machine learning (ML) model (Random Survival Forest - RSF) and a non-ML model (Fine-Gray Model - FGM) were developed and compared to the MAGGIC risk score, which is widely used to predict HF outcomes.
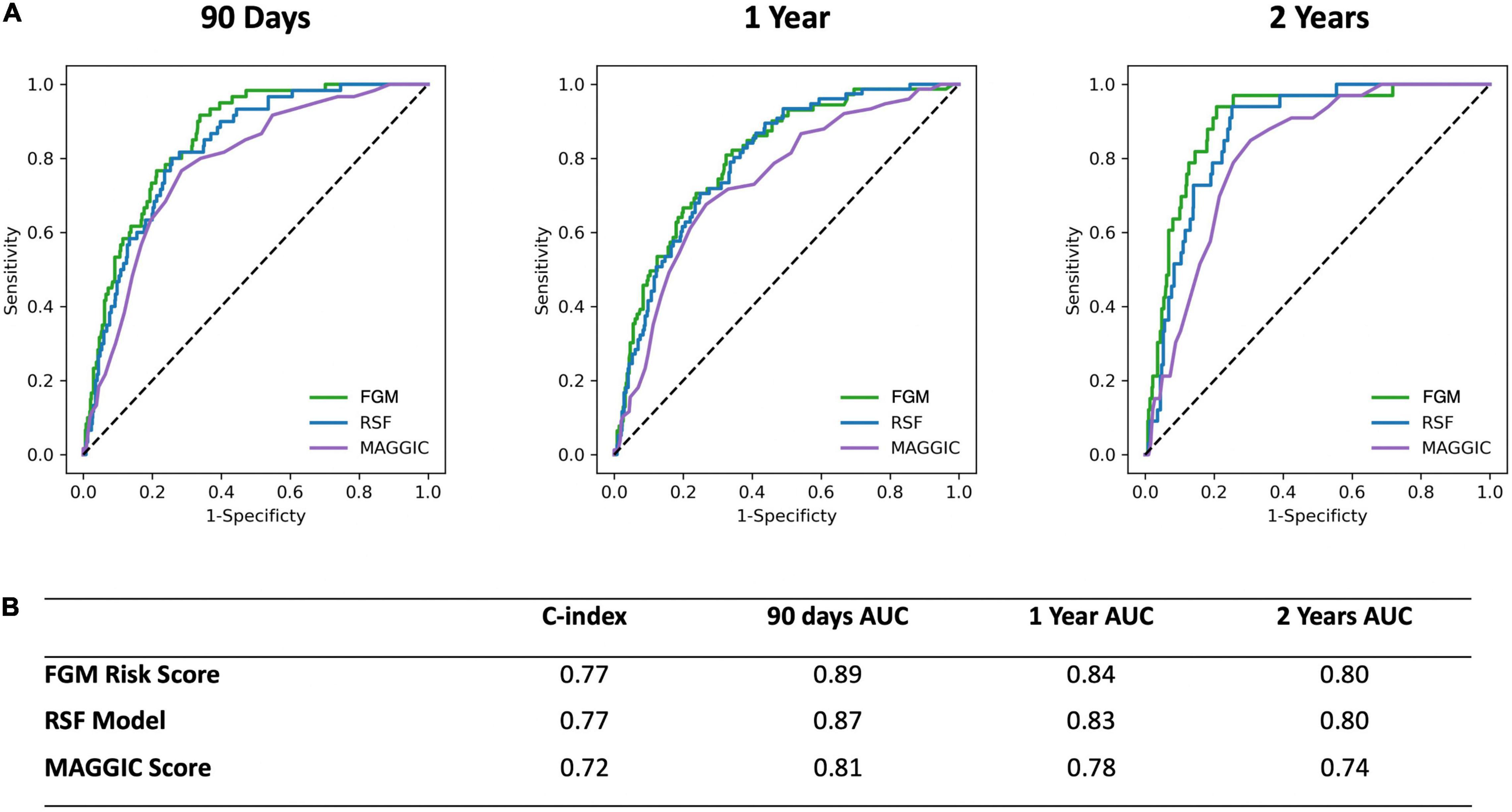
Figure 1 ROC curves for the three predictive models.
Both the FGM and RSF models outperformed the MAGGIC Score for all three time horizons evaluated in the study (Figure 1).
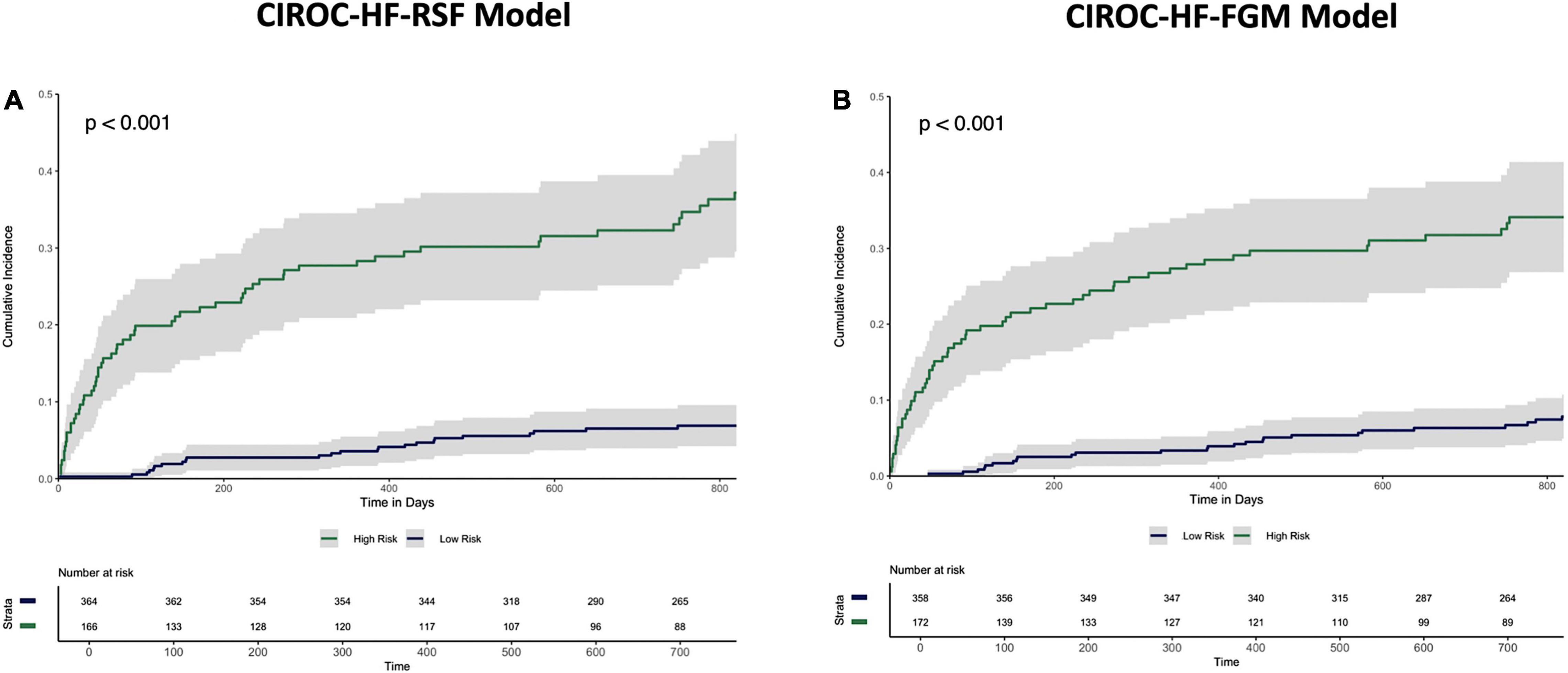
Figure 2 Cumulative incidence curves for time to hospitalization in the holdout dataset in patients stratified as “high-risk” vs “low-risk” in the two CIROC-derived models.
The high risk group had a 34-fold greater risk of HF hospitalization compared to the low risk group at 90 days (Figure 2). This stratification could potentially be used to identify patients who would benefit from care in a dedicated HF program post-discharge to reduce the risk of re-admission.
"Overall, our study supports strong value provided by the routine capture of multi-domain health data resources at time of diagnostic imaging, this approach facilitating the implementation of personalized outcome prediction."
Collaboration
This study utilized data from the Cardiovascular Imaging Registry of Calgary (CIROC), a clinical outcomes registry established at the Libin Cardiovascular Institute by Dr. James White, Cohesic’s CMO and co-founder. Cohesic and the Libin Cardiovascular Institute collaborate on CIROC including development of data science infrastructure and validation of predictive models for potential commercial use. Data were collected between February 2015 and October 2019 at the Stephenson Cardiac Imaging Centre which employs Cohesic’s cardioDI software for workflow, data collection, and clinical reporting. Patient-reported measures of health, as well as research consent, were collected using Cohesic’s intakeDI software.
1Tsao CW, et al. Heart Disease and Stroke Statistics - 2023 Update: A Report From the American Heart Association. Circulation. 2023;147:e93–e621. DOI: 10.1161/CIR.0000000000001123
2Savarese G, et al. Global burden of heart failure: a comprehensive and updated review of epidemiology. Cardiovascular Research, Volume 118, Issue 17, December 2022, Pages 3272–3287. https://doi.org/10.1093/cvr/cvac013
3Cornhill AK, et al. Machine Learning Patient-Specific Prediction of Heart Failure Hospitalization Using Cardiac MRI-Based Phenotype and Electronic Health Information. Front. Cardiovasc. Med., 16 June 2022, Sec. Cardiovascular Imaging, Volume 9 - 2022 https://doi.org/10.3389/fcvm.2022.890904